Enhance Viewer Engagement Through Data Analysis
In an era where content is king, capturing and maintaining viewer engagement can feel like navigating through a crowded, noisy market. How does one manage to not only grab attention but also sustain it? Enhancing viewer engagement through meticulous data analysis provides the beacon needed to light the way. This article discusses how leveraging specific data analytical techniques can significantly improve viewer engagement.
Understanding Viewer Engagement
Viewer engagement is more than just counting views or likes. It involves measuring how viewers interact with content, including time spent, comments, shares, and the quality of these interactions. High engagement rates generally indicate that content is resonant, compelling, and valuable to the audience. For instance, a study by Microsoft highlighted an increase in digital content consumption, yet the human attention span has reportedly declined to just eight seconds. This dichotomy underscores the challenge content creators face: deliver impactful content quickly and effectively.
The Role of Data Analysis in Engagement
Data analysis plays a pivotal role in understanding how, when, and why engagement happens. By analyzing viewer data, content creators can tailor their offerings to meet the specific needs and preferences of their audience. Analytics tools such as Google Analytics, Tableau, or even specialized platforms like Heap and Mixpanel can provide insights into viewer behavior patterns, allowing creators to make informed decisions about content strategies.
Segmentation and Personalization
One effective strategy for enhancing engagement is segmentation followed by personalization. Segmentation involves categorizing the audience based on various criteria such as age, location, and viewing habits. According to a report from HubSpot, personalized call-to-actions have a 202% higher conversion rate than generic ones. By segmenting the audience, content creators can design personalized experiences tailored to different viewer segments, significantly boosting engagement levels.
Content Optimization Through A/B Testing
A/B testing is another powerful tool afforded by data analysis. This involves comparing two versions of a piece of content to see which one performs better on predefined metrics such as views, average watch time, and interaction rate. This method was notably used by Netflix, which regularly utilizes A/B testing to optimize choices of thumbnail images – resulting in a reported increase in engagement up to 30%. Through continuous A/B testing, content creators refine their strategies, ensuring the content resonates well with their audience.
Timing and Scheduling Strategies
The timing of content release can significantly influence viewer engagement. Analysis of engagement patterns can help pinpoint the best times for posting content, ensuring it reaches the maximum number of viewers. For instance, a study by Sprout Social found that posts published on Wednesdays between 11 a.m. and 1 p.m. got the most engagement. Employing an effective scheduling strategy based on viewer data can help maximize visibility and engagement.
Feedback Loops and Continuous Improvement
Data analysis also enables the establishment of feedback loops, where viewer responses can be used to continually refine and improve content. Engaging directly with comments or adjusting content based on feedback are effective ways to build a loyal audience. For example, YouTube provides extensive analytics on viewer demographics, playback locations, and engagement metrics, allowing creators to continuously adapt and enhance their content strategies.
Case Studies: Success Stories
Several companies have thrived by leveraging data analysis for viewer engagement. BuzzFeed, for example, owes much of its success to its data-driven approach to content creation and distribution. By analyzing which types of content received more engagement, BuzzFeed could tailor its articles, videos, and quizzes to better suit viewer preferences, thereby maintaining high engagement rates across platforms.
Another example is Twitch, a popular streaming platform, which uses data analytics to offer personalized game recommendations, optimize stream times for its users, and enhance user interaction through chat optimizations. Such strategic use of data has helped Twitch become one of the most engaged platforms in the gaming community.
Conclusion: The Future of Engagement
Enhancing viewer engagement through data analysis isn’t just a strategy for today—it’s a staple for the future of content creation. As technology evolves and more sophisticated tools become available, the depth and breadth of data will increase, providing even richer insights into viewer preferences and behavior. The success lies in how well content creators can use this data to deliver personalized, timely, and impactful content that keeps viewers coming back for more. Thus, the mantra for success in the digital content arena boils down to this: analyze, personalize, and engage.
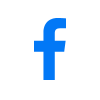
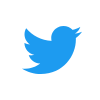
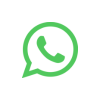
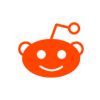
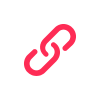
Recent Comments